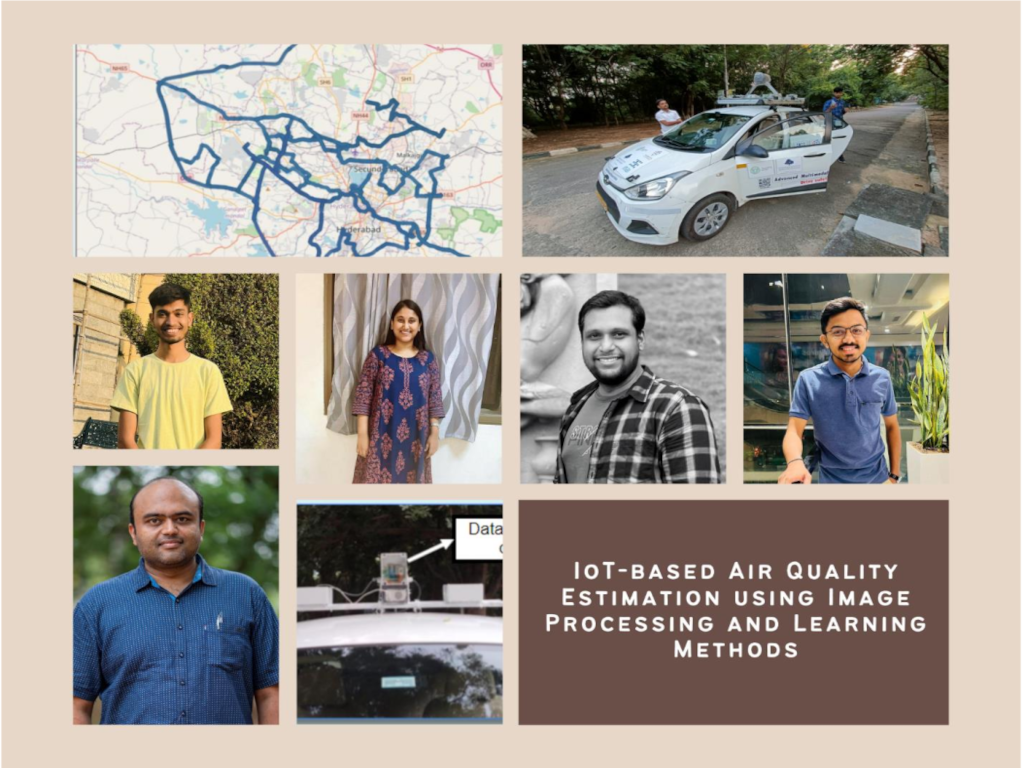
Air Quality Index
Air pollution is a concern for the health of all living beings. It is essential to check on the quality of air in the surroundings. In an effort to develop a more efficient and cost-effective air quality monitoring solution, the team of Prof. Sachin Chaudhari, Nitin Nilesh, Spanddhana Sara, Om Kathalkar, Shreyash Gujar worked to provide an IoT-based real-time air quality index (AQI) estimation technique using images and weather sensors in the Indian city of Hyderabad.
The IIITH team, in collaboration with the Society for Cyberabad Security Council and the State Government of Telangana, embarked on the AirIoT project to monitor air pollution levels in the Gachibowli area. Despite deploying 40-50 air pollution monitoring devices in the area, the team encountered a major hurdle due to the limited shelf life of conventional IoT sensor devices and had to replace at least 100 more of the original devices due to their high rate of failure. This setbackprompted the team to seek a more sustainable approach to air pollution monitoring that could be scaled up at the city level.
The project was titled “IoT-based Air Quality Estimation using Image Processing and Learning Methods”
To address this challenge, the team developed a low-cost, sustainable air quality monitoring system using machine learning algorithms and edge computing. The new system uses a combination of sensors and cameras to collect data on air pollution levels and then processes the data in real-time using machine learning algorithms to generate insights and alerts. The system also leverages edge computing to minimize the need for data transfer to the cloud, thereby reducing costs and improving efficiency.
The project was titled “IoT-based Air Quality Estimation using Image Processing and Learning Methods” and relied on deep learning algorithms and image processing techniques, which required fewer resources and less manpower compared to conventional methods.
To develop this new AQI estimation method, the team utilized a combination of image features such as traffic density and visibility obtained from a camera mounted on top of the Bodhayan Car, and sensor features such as temperature and humidity obtained from nearby weather stations. The team then utilized object detection and localization-based deep learning techniques, along with image processing methods, to extract the necessary features for AQI estimation.
To train the machine learning model to estimate AQI, the team created a dataset of images with co-located AQI values collected across different seasons, by driving the Bodhayan Car on the streets of Hyderabad. The front-facing camera on the car was trained with air pollution data captured via the sensors, and the ML model was trained to categorize air pollution into 5 different classes - Good, Satisfactory, Moderate, Poor, and Severe, making it more user-friendly than continuous numbers.
The team reported an 82% accuracy in estimating AQI levels using this image- based method, and when compared with existing research studies on image- based AQI detection, this method showed an improvement in the accuracy of AQI estimation. By providing more accurate and real-time data on air quality, policymakers can make informed decisions and take effective measures to improve air quality and protect public health. This new approach to AQI estimation has the potential to revolutionize the way air quality monitoring is conducted, and can help cities worldwide in their efforts to address the critical issue of air pollution.