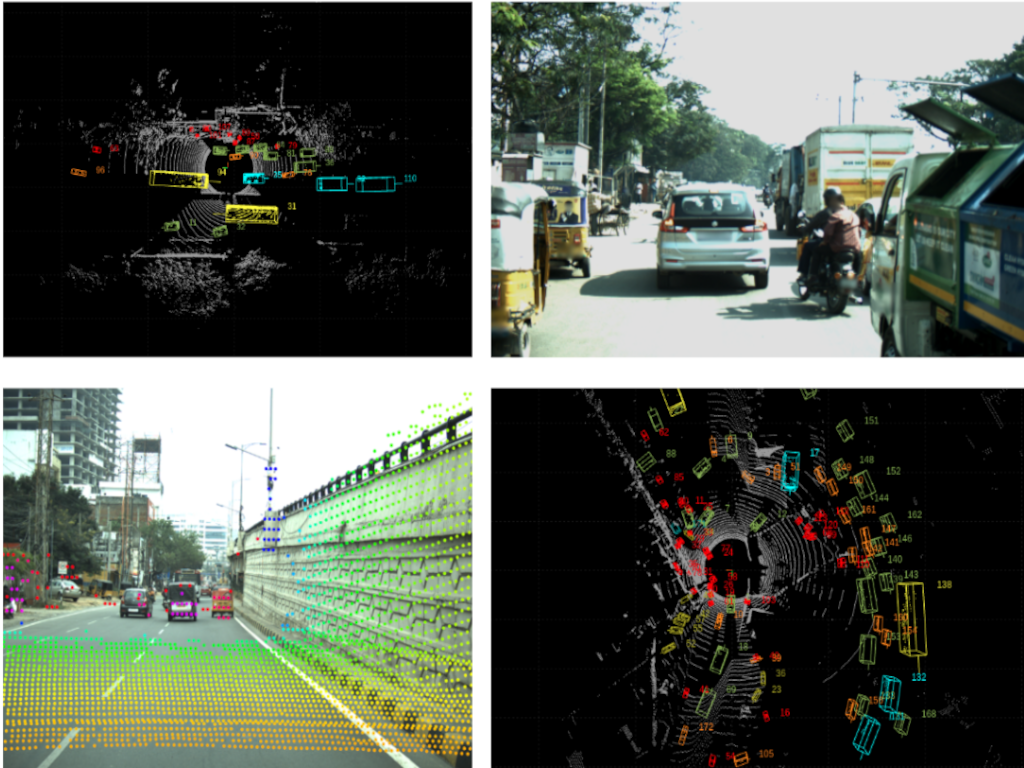
IDD-3D: Indian Driving Dataset for 3D Unstructured Road Scenes
Autonomous driving technology has made significant strides over the past decade, but most advancements have been tailored to structured environments. However, the real world is far from structured; it's chaotic, unpredictable, and diverse. This is where IDD-3D comes into play. This groundbreaking dataset aims to bridge the gap by providing a comprehensive collection of unstructured driving scenarios, enabling more robust and adaptable autonomous driving systems.
Problem Space & IDD-3D
Existing datasets for autonomous driving often fall short in capturing the complexity and unpredictability of unstructured driving scenarios. They are usually limited to controlled and well-structured environments, which are not representative of real-world conditions. Enter IDD-3D, a dataset designed to tackle these challenges head-on.
Shubham Dokania, A.H. Abdul Hafez, Anbumani Subramanian, Manmohan Chandraker, C.V. Jawahar
IDD-3D stands out for its meticulous data acquisition process, capturing over 5 hours of driving data in diverse regions of Hyderabad, India (Refer to Fig.2). The dataset includes detailed 3D bounding box annotations across 17 categories, providing a rich resource for object detection and tracking (Refer to Fig. 3). Moreover, it offers a class-wise distribution of common objects like cars, pedestrians, and more, making it invaluable for understanding traffic and crowd density (Refer to Fig. 4).
Experimental Results Summary, Interesting Cases, and Applications
The paper presents an extensive analysis of IDD-3D through various experiments. It shows that popular methods like CenterPoint, SECOND, and PointPillars perform consistently across different object categories (Refer to Tables 2, 3). The dataset also includes a 3D object tracking evaluation, providing valuable insights into object motion and behavior (Refer to Table 5 ).
IDD-3D captures a range of interesting and safety-critical scenarios, such as jaywalking pedestrians and high-density traffic, which are often overlooked in existing datasets. These features make IDD-3D highly applicable in fields beyond autonomous driving, including road safety, surveillance, and traffic management.
Conclusions
IDD-3D is a significant leap forward in the quest for safer and more reliable autonomous driving systems. By capturing the intricacies of unstructured driving scenarios, it provides a robust foundation for future research and applications. As autonomous driving technology continues to evolve, datasets like IDD-3D will play a pivotal role in shaping a future where cars can navigate the world as adeptly as humans.
Paper:
Project Website
https://idd3d.github.io ((website not complete yet))