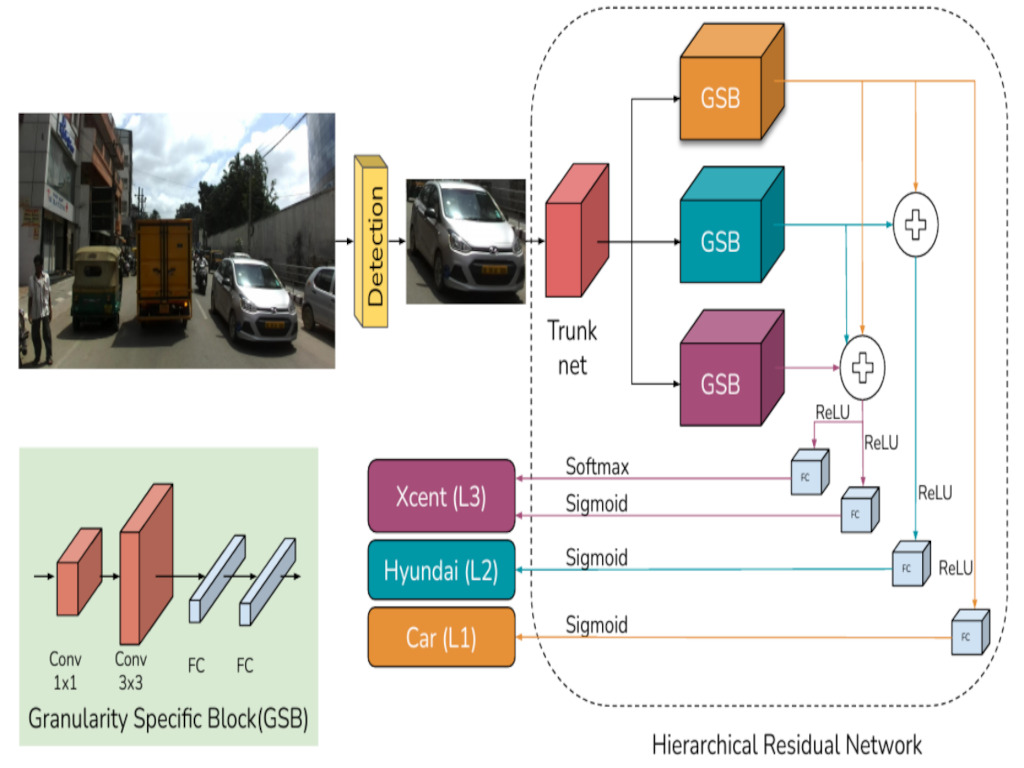
A Fine-Grained Vehicle Detection (FGVD) Dataset for Unconstrained Roads
Intelligent traffic monitoring systems are of utmost need in big cities for public security, planning, and surveillance. The detectors used for vehicle re-identification in on-road surveillance systems should finely classify the vehicle’s type, manufacturer, and model for detailed analysis and feedback. However, existing detection datasets only contain information regarding the vehicle’s type (eg. car, motorcycle, etc.). Enter FGVD, the first Fine-Grained Vehicle Detection dataset comprising 210 unique fine-grained labels (with manufacturer eg. Honda, Bajaj, etc. and model information for eg. Ciaz, Pulsar150, etc.) of multiple vehicle types organized in a three-level hierarchy.
The FGVD dataset includes 24450 vehicle bounding boxes annotated in 5502 scene images captured from a moving camera mounted on a car. The dataset is challenging as it has vehicles in complex traffic scenarios with intra-class and inter-class variations in types, scale, pose, occlusion, and lighting conditions. Moreover, the dataset distribution is naturally long-tailed due to the infrequent occurrence of various vehicle models.
FGVD Model Architecture & Experimental Results:
Current object detectors like yolov5 and faster RCNN perform poorly on this dataset due to a lack of hierarchical modeling between the coarse-level (vehicle type) and fine-grained labels (manufacturer and model). This paper additionally presents a customized model combining existing detectors and the Hierarchical Residual Network (HRN) classifier tailored for the FGVD task.
The paper showcases the detection results of the YOLOv5l+HRN model on the FGVD dataset. The figure below shows the qualitative results of the proposed FGVD model. Refer to the paper for a detailed quantitative analysis of the model’s performance.
The interpretability of the FGVD model when detecting different label hierarchies is shown in the GradCAM visualizations shown below.
Conclusion:
The paper presents the first dataset for the Fine-Grained Detection of Vehicles while also providing a customized model for this task that outperforms existing detectors by a large margin across all label hierarchies (vehicle's type, manufacturer, and model). The FGVD dataset can potentially be useful for vehicle re-identification in on-road surveillance systems, generating alarms for road safety systems, and promoting the development of ADAS products for Indian roads.
Paper Link: https://dl.acm.org/doi/10.1145/3571600.3571626
Dataset Link: https://zenodo.org/record/7488960
GitHub Repo: https://github.com/iHubData-Mobility/public-FGVD
The project is funded by the iHub-Data and Mobility at IIIT Hyderabad. We thank the data collection and annotation team for their effort.