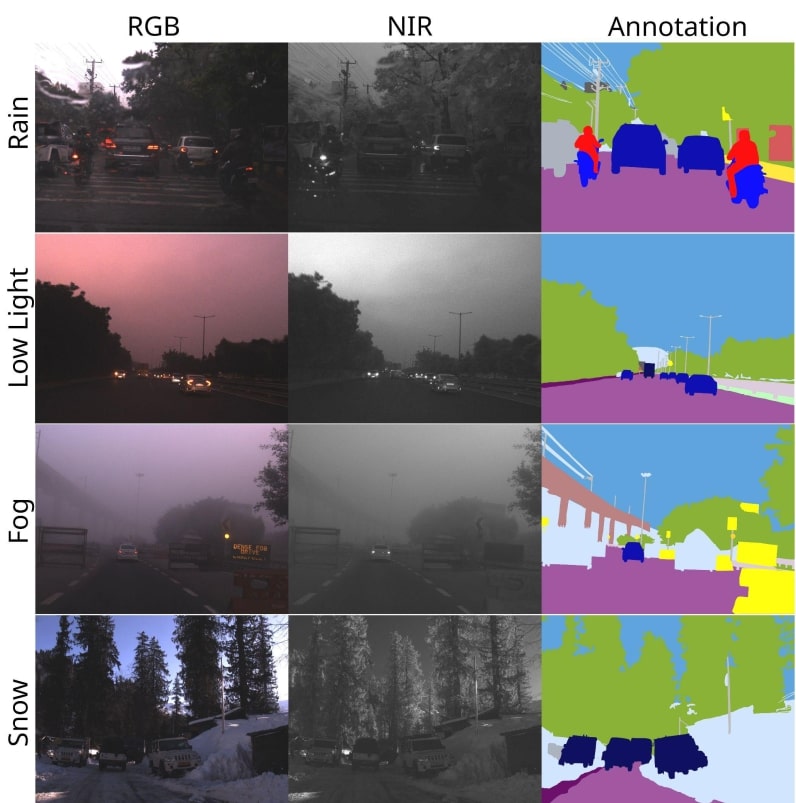
IDD-AW: A Benchmark for Safe and Robust Segmentation of Drive Scenes in Unstructured Traffic and Adverse Weather
IDD-AW is a groundbreaking dataset designed to address the challenges of autonomous driving in adverse weather conditions and unstructured environments. While existing datasets have primarily focused on well-organized, controlled settings, IDD-AW takes a different approach by capturing the complexities of real-world driving scenarios. The dataset is particularly unique for its focus on adverse weather conditions like rain, fog, snow, and lowlight, collected in cities all across India.
Key Features:
- Diverse Geographical Coverage: IDD-AW is collected across various states and terrains of India, from the highways of Hyderabad to the snowy hills of Manali and the foggy roads of Delhi and Ooty encompassing a range of road types, traffic densities, and adverse weather conditions like rain, fog, snow and lowlight.
- Rich Annotations: The dataset includes both NIR and RGB paired components for each image and is annotated for semantic and instance segmentation.
- High-Quality Data: The dataset is meticulously curated, with high-resolution RGB and NIR camera sensors capturing over 1 million frames. However, through thorough inspection and high-quality checks, the final dataset has shortlisted 5000 RGB-NIR image pairs.
- Unique Object Categories: Unlike other datasets that often generalize objects into broad categories, IDD-AW provides a more nuanced classification, including unique vehicle types and pedestrian behaviors commonly seen in unstructured environments and adverse weather conditions.
We also introduce a novel evaluation metric, "Safe mIoU," we address the limitations of traditional mIoU metrics by penalizing risky mispredictions. This metric is particularly useful for hierarchical datasets, where penalty severity is determined by tree distance. We apply this metric across diverse label sets, ranging from comprehensive sets to specific classes such as traffic participants and roadside objects—crucial for autonomous driving applications.
Dataset Characteristics:
IDD-AW is split into four sets corresponding to the examined conditions. The dataset consists of 1500 rainy, 1500 foggy, 1000 lowlight, and 1000 snowy images from the recordings for dense pixel-level semantic annotation, for a total of 5000 adverse-condition images. We use the same set of labels that are used by IDD. This label set has multiple levels of hierarchy ranging to four levels with level 1 having 7 labels to level 4 having 30 labels.
Comparison of ACDC & IDD-AW: Pixel wise (left), traffic participants instances count (right). Pixel counts are normalized by resolution and the number of images in the datasets. IDD-AW has more pixels (left) as well as more instances per image (right) of traffic participants (TP) which includes all vehicles and living things that represent unstructured traffic. Note that there are over 300 images in IDD-AW with more than 20 instances of TP while ACDC has only around 10.
Safe mIoU:
Traditional mIoU is a widely used metric for semantic segmentation but falls short in assessing the safety of driving scenes and in treating all classes equally. To address this, we propose “Safe mIoU (SmIoU)”, a refined metric considering the severity of misclassifications in critical classes (e.g., pedestrians, vehicles). SmIoU introduces a hierarchical penalty based on semantic relationships, penalizing misclassifications based on their distance in the class hierarchy. The final score is computed by taking the mean of IoUs with penalties, providing a holistic evaluation aligning with driving scene priorities and safety considerations.
Experimental Analysis:
Experimental Results and Analysis:
Qualitative examples from each condition with predictions using models trained on ACDC, IDD, and IDD-AW Datasets and the ground truth at the end
Conclusions:
We present IDD-AW, a dataset for challenging driving scene understanding in adverse weather conditions and unstructured traffic. We also introduce a new metric “Safe mIoU”, a metric incorporating safety into segmentation evaluation. Benchmarking state-of-the-art models revealed differences between traditional mIoU and Safe mIoU, emphasizing critical class distinctions and how Safe mIoU is a better metric for measuring the safety of a trained model in segmentation. Future work includes exploring more efficient loss functions for optimizing safe mIoU.
Note:
This work was done in collaboration with the iHub team at IIIT-H and DYSL-AI Lab at DRDO.