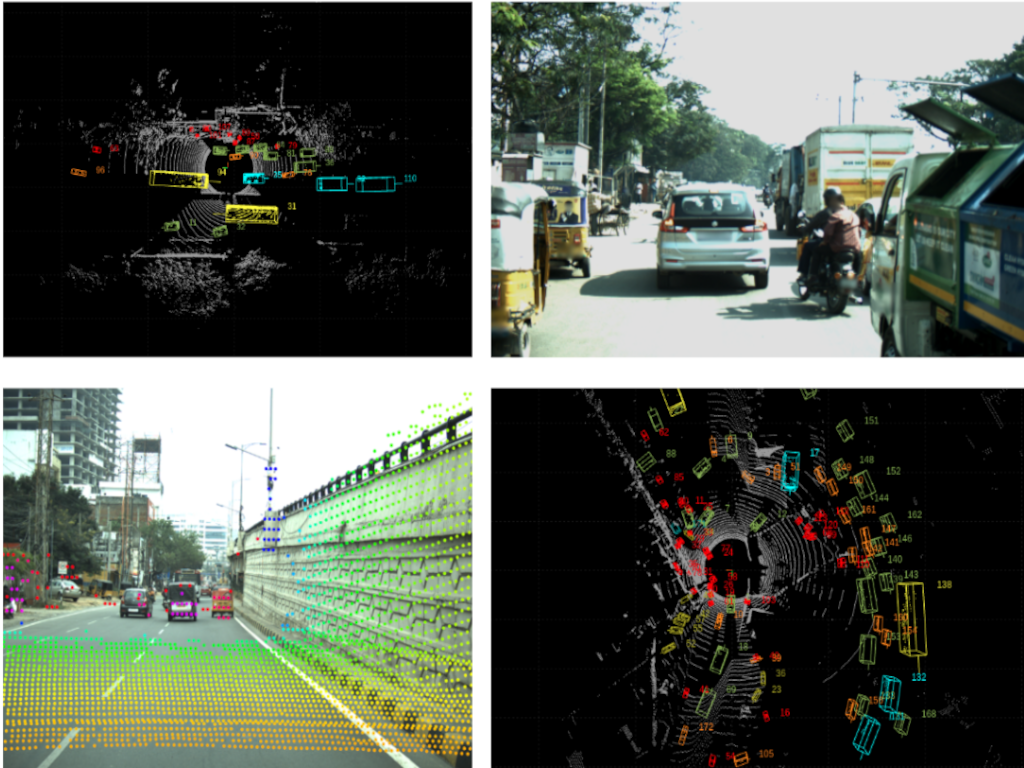
Making Roads Better, One Traffic Sign at a Time!
Road accidents affect millions of lives due to increasing population and vehicle density, and incorporating Advanced Driving Assistant Systems (ADAS) in commercial vehicles is gaining momentum. However, these systems still have a long way to go regarding offering absolute road safety. In a recent road safety report, it is observed that 12.6% of accidents caused by driver errors are due to traffic sign violations, which establishes the importance and need for traffic signs in a regulated manner. Autonomous Vehicles (AV) require a consistent and well-maintained infrastructure to explore their full potential and deliver the safety they promise. Traffic signs are an important aspect of road infrastructure, as they contain essential information about what is coming ahead, which ADAS systems like Mobileye use as supplementary information for scene understanding. Failure to robustly perceive and process the road scene has led to multiple fatal crashes involving commercially deployed AVs as well.
Gupta, Varun, Anbumani Subramanian, C. V. Jawahar, and Rohit Saluja. "CueCAn: Cue Driven Contextual Attention For Identifying Missing Traffic Signs on Unconstrained Roads." International Conference on Robotics and Automation (2023).
Thus, we can conclusively establish the need for a good road infrastructure, of which road signs are an essential aspect. This is what our team from CVIT, IIIT Hyderabad, planned to address and improve.
It is essential to understand what is meant by traffic sign context, which is simply the visual information or a hint with which a traffic sign can be recognized without it being present in the scene. For instance, it is the bend in the road towards the left for a left-hand curve sign. With the help of an in-house data-collection and annotation team, we collected videos in and around Hyderabad in diverse conditions to make the resulting solutions robust to as many artifacts as possible. We call this dataset the Missing Traffic Signs Video Dataset (MTSVD), which has dense annotations for the traffic signs present on the road and the ones that remain absent. For the traffic signs on the road, bounding box and track annotations are provided with multi-label attribute markings, resulting in 10K+ tracks and 1.2M+ frames.
What makes this task quite interesting is the idea of identifying something Missing! Creating supervision labels is a challenge as well. Specifically with traffic signs, the videos are recorded from a dashcam PoV, and to manually add bounding box annotations for missing signs is a road (no puns intended) to disaster, as human biases in scale, size, etc. get involved. So for this, we came up with an interesting approach of using image inpainting to ‘remove’ existing traffic signs, as if they were missing from the scene, now having exact coordinates of where and in what dimension they were present in the actual scene, and the solutions were tested on a mix of such inpainted scenes, and scenes with actual missing traffic signs.
Along with the dataset, MTSVD, we introduced attention units, CueCAn (Cue-Driven Contextual Attention Units), to help the encoders emphasize and focus on the traffic sign cues. While creating this module, the idea was based on an observation that contextual cues for traffic signs add some discontinuity in the scene, and cohesively identifying this discontinuity pattern in many images would provide the needed supervision for the models to look at the cues of the traffic signs better. CueCAn units significantly improved the model’s ability to focus on the traffic signs cues, which we corroborated with GradCAM visuals. The overall approach is briefly illustrated in the visual below.
Scenes with real and inpainted traffic signs (chevron-left). Middle: Intermediary GradCAM visualizations of the cue classifier (encoder) with and without CueCAn.Right: Segmentation model with CueCAn-based encoder detects missing signs (green masks overlayed over the scene on the right for CueCAn and yellow mask by the baseline) on the scene without symptoms (follow pink arrows) by effectively attending to the context cues, compared to weak attention without CueCAn. Segmentation GradCAMs are obtained from the centroid of the predicted sign (red dot).
For more details, I strongly encourage you to read the paper,
Gupta, Varun, Anbumani Subramanian, C. V. Jawahar, and Rohit Saluja. "CueCAn: Cue Driven Contextual Attention For Identifying Missing Traffic Signs on Unconstrained Roads." International Conference on Robotics and Automation (2023).
The dataset is made public and is available to be downloaded from https://idd.insaan.iiit.ac.in/
The project is funded by the iHub-Data and Mobility at IIIT Hyderabad. We thank the data collection and annotation team for their effort.